MASt Thesis - Metric tomography with Sobolev gradients
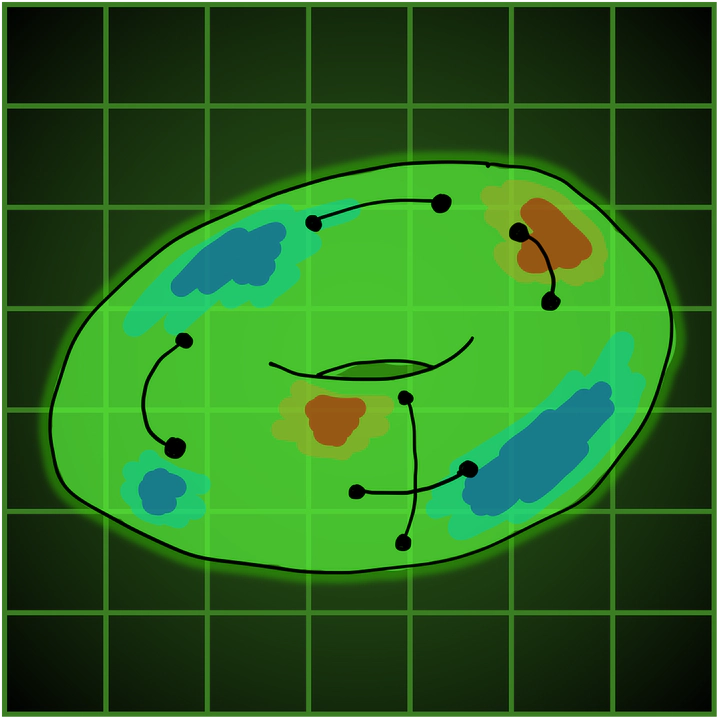
For the general public (click to open and close)
Even though the ‘metric tensor’ seems like an exotic object that only exist in general relativity, it’s actually just a tool to describe effective distances. For example, it could be used to describe time that sound waves take, like inside of the Earth! This could be anisotropic, meaning that sound could be faster in one direction than the other. The speed of sound waves are determined by a myriad of factors, such as density and composition.
The next (practical) question is, how do we determine the composition given that we have measured some sound waves at certain locations? Given a metric tensor, we have the ‘forward’ equation that predicts how sound waves will travel, but now we are describing an ‘inverse’ problem where we want to determine the metric tensor with essentially infinite parameters given some finite measurements. How do we do this? A basic way to do this is to use finite parameterisation and use regularisation to constrain the model. A better way is to work in function space and calculate functional derivatives, then do gradient descent. Practically speaking, we resolve them via Fourier kernels, but this is fine, since after this we can increase the number of Fourier kernels until the solution converges.
We want to impose any assumptions/knowledge we have about the solution. For example, point-wise convergence and some levels of differentiability are probably good assumptions. To constrain solutions to have such properties, we can work in specific functions spaces – Sobolev spaces are such spaces.
Abstract (click to open and close)
In underdetermined inverse problems such as ray-tracing tomography, finite-parametrisation of the model and regularisation of the misfit functional are often used to force a unique solution. However, these measures are unsatisfactory because they are arbitrarily chosen. An alternative method is to use gradient-based optimisation to find a local minimum of the misfit, where the first order gradient of the misfit is required for the scheme and the second order can be used to quantify the constraints on the obtained solution or to potentially improve the scheme. The adjoint method is an effective way of calculating these gradients. We show that, in order to obtain a sensible solution through this scheme, we must work in an appropriate Sobolev space, the space of square-integrable functions with additional continuity constraints. We apply these ideas to the metric tomographic problem, the problem of finding an unknown metric on a manifold given a finite number of geodesics, which is an analogous problem to ray-tracing tomography of surface waves. We numerically implement this method for the linearised version of the problem on a 2-dimensional torus. Additionally, we present the first and second adjoints for the general problem, which can be used to calculate the first and second order gradients of the misfit function.